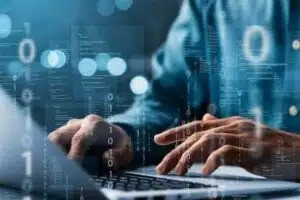
Businesses are continuously seeking innovative ways to manage and utilize their data efficiently. One such solution that has gained significant traction is the data lake architecture. This modern approach to data storage and analysis enables organizations to handle large volumes of diverse data types, making it an essential component of today's data strategy. In this article, we'll explore what data lake architecture entails, its core components, the benefits it offers, the challenges it presents, and best practices for implementation.
What is Data Lake Architecture?
Data lake architecture is a storage system designed to hold vast amounts of raw data in its native format until it is needed for analysis. Unlike traditional databases and data warehouses that require data to be structured before storage, data lakes can accommodate structured, semi-structured, and unstructured data. This flexibility allows businesses to store data from various sources without the need for extensive preprocessing. Essentially, a data lake acts as a centralized repository where data can be ingested, stored, and processed as required.
Core Components of Data Lake Architecture
The foundation of data lake architecture is built on several key components that work together to manage data efficiently. First is the ingestion layer, where data from different sources such as databases, IoT devices, and social media is collected. Next is the storage layer, typically implemented using scalable storage solutions like Hadoop Distributed File System (HDFS) or cloud-based storage services. The processing layer, which includes tools like Apache Spark and Flink, allows for the transformation and analysis of data. Finally, the access and security layer ensures that data governance, compliance, and access controls are maintained, safeguarding sensitive information.
Benefits of Data Lake Architecture
Adopting a data lake architecture offers numerous advantages for businesses. One of the primary benefits is its scalability, enabling organizations to store and manage large datasets without worrying about capacity constraints. The ability to store data in its raw form also means that companies can retain all information, which can be useful for future analysis or unforeseen use cases. Furthermore, data lakes support advanced analytics and machine learning by providing a vast pool of data that can be leveraged to uncover insights and drive innovation. This holistic approach to data management enhances decision-making and fosters a data-driven culture within the organization.
Challenges and Considerations
- Drama at City of Harare bosses trial
- Price of bread skyrockets again
- Price of bread skyrockets again
- ‘Zim’s retailers deserve a wage subsidy’
Keep Reading
While data lake architecture presents many benefits, it also comes with its set of challenges. One of the most significant issues is data quality, as storing raw data can lead to inconsistencies and inaccuracies if not properly managed. Additionally, the sheer volume of data can make it difficult to locate and retrieve specific information, leading to what is often referred to as a "data swamp." Ensuring robust data governance and implementing efficient metadata management practices are crucial to addressing these challenges. Moreover, organizations must invest in the right tools and expertise to effectively manage and secure their data lakes, protecting them from unauthorized access and breaches.
Best Practices for Implementing Data Lake Architecture
To maximize the benefits of data lake architecture, businesses should follow several best practices. Firstly, it's essential to establish clear data governance policies to ensure data quality and consistency. Implementing a robust metadata management system helps in cataloging and indexing data, making it easier to locate and use. Organizations should also focus on building a scalable infrastructure that can grow with their data needs. Regularly auditing and monitoring the data lake ensures compliance with regulatory requirements and helps in identifying any potential security threats. Finally, fostering a culture of collaboration between data engineers, analysts, and business stakeholders is vital for the successful adoption and utilization of data lakes.
Future Trends in Data Lake Architecture
As technology continues to evolve, so too does the landscape of data lake architecture. One emerging trend is the integration of artificial intelligence (AI) and machine learning (ML) directly into data lake platforms. This integration allows for real-time analytics and automated insights, enabling businesses to make faster and more accurate decisions. Additionally, the rise of multi-cloud and hybrid cloud environments is influencing the way data lakes are deployed and managed. Organizations are increasingly adopting these flexible architectures to avoid vendor lock-in and to leverage the best tools and services available across different cloud providers. These trends highlight the dynamic nature of data lake architecture and its capacity to adapt to the ever-changing needs of the business world.
Real-World Applications of Data Lake Architecture
Data lake architecture is being utilized across various industries, showcasing its versatility and effectiveness. In healthcare, for example, data lakes are used to aggregate and analyze patient data from multiple sources, improving diagnostics and personalized treatment plans. In the financial sector, data lakes help in fraud detection and risk management by providing comprehensive data analysis capabilities. Retailers use data lakes to understand customer behavior and optimize inventory management, while the entertainment industry leverages them to analyze audience preferences and enhance content delivery. These real-world applications demonstrate the broad potential of data lake architecture to transform data into actionable insights, driving value across different sectors.
Data Lake Architecture
Data lake architecture represents a transformative approach to data management, offering unparalleled flexibility and scalability for organizations dealing with diverse data types. By understanding its core components, benefits, and challenges, businesses can effectively leverage data lakes to enhance their data strategy. Implementing best practices ensures that data lakes remain a valuable asset, driving innovation and informed decision-making. As the digital landscape continues to evolve, data lakes will undoubtedly play a pivotal role in helping companies navigate and harness the power of big data.